Harnessing the Power of Generative AI in Financial Services
From engaging with customers to enhancing fraud prevention controls to streamlining mundane compliance activities, generative artificial intelligence (AI) tools can benefit companies across all industries in boundless ways. At the same time, generative AI also has the potential to introduce unintended risks, absent proper policies and procedures and robust internal controls.
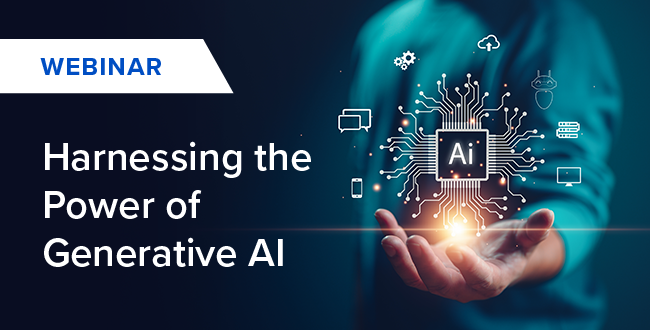
In our recent webcast, “Harnessing the power of generative AI in financial services,” experts discussed the current state of generative AI adoption today, the opportunities and risks that its use creates, particularly in the financial services industry, as well as emerging best practices around the adoption of generative AI tools.
Current state of AI adoption
A webcast poll conducted by Smarsh revealed that firms are at various levels of maturity concerning their use of AI-enabled tools. From our audience survey, we found that:
- Nearly 27% of respondents said they are piloting AI-enabled tools in limited capacity
- 25% said they are prohibiting the use of AI altogether until the risks are assessed
- Another 22.5% of respondents said they’re assessing the use of AI for specific apps
- Nearly 17% said they are currently performing due diligence on existing tools
- Just 9% said they have deployed generative AI enterprise-wide
“At a high level,” said Tiffany Magri, senior regulatory advisor at Smarsh, “the findings indicate that many firms today are making efforts to understand how these AI tools work, set the appropriate guardrails, and to better understand where their compliance and regulatory obligations lie.”
Jake Frasier, senior manager at FTI Consulting, said the findings align with what he has been hearing anecdotally from chief compliance officers. Oftentimes, firms will put out a policy prohibiting the use of AI tools for certain applications, “while also conducting due diligence and working on what that policy is going to look like in the future,” Frasier said.
Generative AI use cases
In financial services, common use cases for generative AI from a compliance and risk management standpoint include:
- AI-matched contextual documents
- AI-powered research retrieval
- AI-modeled risk scenarios
More specifically, today’s advanced AI tools can recognize handwriting, speech, and images instantaneously and seamlessly and also can be used for reading comprehension and translating documents in foreign languages.
“There are just so many different uses and applications for these technologies that can be used within your firm to make some of these [compliance] processes less manual and provide those efficiencies that you’re really looking for, especially for overwhelmed compliance departments,” Magri said. “Being able to make some of these very time-consuming tasks more manageable and ultimately a lot more effective is always a great thing for compliance officers.”
Firms begin to raise their regulatory risks exponentially when they start using capabilities like robo-advisers — which provide algorithm-driven financial advice — and automated chatbots in responding to client inquiries.
“For example,” Magri said, “we’ve seen chatbots that provide basic question-and-answering, and all the way up to providing investment advice. Being able to use some of this technology is amazing, but you have to make sure you have the proper guardrails in place.”
Generative AI compliance implications
The proliferation of digital communication channels today exacerbates the risks posed by generative AI, which has resulted in a major Big Data problem. “Firms are dealing with a very large, heterogeneous set of information that has very high velocity and a significant amount of veracity. Communication formats are very different,” said Robert Cruz, vice president, information governance for Smarsh.
“You need a clean, well-managed set of information to effectively leverage these [AI] models to their fullest,” Cruz added.
Microsoft Teams, for example, offers a variety of features — such as whiteboard sharing and voice and video chats — which is an entirely different makeup than other communication tools, like Slack, Zoom, or WhatsApp. “These are the things that companies are leveraging for business,” Cruz said.
The use of all these digital communication platforms, combined with all their various data formats and with a multitude of capabilities, is having major implications on compliance departments today.
“It used to be that years ago firms could apply the same policies and procedures and reviews and supervision across the board,” Magri said. That’s no longer the case. “The size and complexity of the data that's coming in has just exploded as we’ve seen in the last couple years,” she said.
Generative AI best practices
If financial services firms are to reap all the benefits that generative AI provides without the risks, it’s essential that they first put in place the proper policies and procedures and robust internal controls.
The following best practices should not be considered after the fact but rather during the planning and implementation stages.
Practice the principle of explainability
Any firm that uses AI must follow the principle of explainability. Explainability is a way to build not only trust with all stakeholders concerning the use of AI-generated data, but also a way to build responsible AI from the get-go, ensuring that it’s used ethically.
As a starting point, firms should consider the following questions:
- Can you interpret and articulate the data?
- Are the inputs and outputs of the data understood, and can they be validated?
- How are the metrics defined that are going to be used to do this?
- How will any potential bias in the data be identified and removed?
“Being able to effectively communicate that explainability firmwide is also crucial,” Magri said. Those using AI should understand the decision-making process in order to build trust and confidence around both the input and output of the AI models.
That’s something to consider as part of the chief compliance officer’s responsibilities as well. “Can [the CCO] understand this and put it into plain English?” Magri said.
It’s okay if the CCO does not understand the tech lingo of the chief data scientist, Frasier noted. In fact, it’s a good barometer, “because that means you don’t have explainability nailed down yet,” he added.
“It’s almost better if you don't quite understand the data science of feature weighting and feature engineering and the math, because you are the check,” Frasier continued. “If you don’t understand it in plain English, then it’s going to be very hard to talk to a regulator or to a court.”
Have a plain English summary readily available
Firms should readily have a plain English, high-level summary of their AI models if they have to explain it to regulators in the event of an investigation, for example. The executive summary should present an overview of the AI model’s functionality, key components and potential risk areas to ensure that stakeholders at all levels, including the board, comprehend the fundamental workings of the AI system. OCC examiners have commented that failure to provide a plain English summary will be perceived as a red flag, indicating potential vulnerabilities or inadequate risk management strategies, Magri said. “So, it’s going to be important to get that right,” she said.
Avoid bias in the data
“I think it’s important to remember that AI, by definition, is biased,” Frasier said. Feature weighting, which involves moving up the weight or down the weight of certain data elements, inherently creates bias in AI models. “It's not the bias itself that's the problem. It’s the harm that would come from that specific bias.”
Take, for example, an AI model that uses historic data to determine the likelihood of a homeowner defaulting on a loan just based on a zip code. If that zip code maps to race, that can potentially result in a violation of the Fair Credit Reporting Act or the Fair Housing Act. In that type of scenario, “it would be important to bring the feature weight of the zip code down to zero,” Frasier explained, so that the zip code is not weighted at all in the AI model.
Never ignore the human component
“A key component of AI is the human component,” Magri stressed. This is something that regulators have been stressing as well and will continue to focus on, “so don’t let that get lost in the AI models,” she said.
Frasier noted that the human component is an important part of avoiding bias in data as well: “There has to be a lot of oversight in creating [AI] models, curating the models, testing the models for bias.”
Share this post!
Smarsh Blog
Our internal subject matter experts and our network of external industry experts are featured with insights into the technology and industry trends that affect your electronic communications compliance initiatives. Sign up to benefit from their deep understanding, tips and best practices regarding how your company can manage compliance risk while unlocking the business value of your communications data.
Ready to enable compliant productivity?
Join the 6,500+ customers using Smarsh to drive their business forward.
Subscribe to the Smarsh Blog Digest
Subscribe to receive a monthly digest of articles exploring regulatory updates, news, trends and best practices in electronic communications capture and archiving.
Smarsh handles information you submit to Smarsh in accordance with its Privacy Policy. By clicking "submit", you consent to Smarsh processing your information and storing it in accordance with the Privacy Policy and agree to receive communications from Smarsh and its third-party partners regarding products and services that may be of interest to you. You may withdraw your consent at any time by emailing privacy@smarsh.com.
FOLLOW US